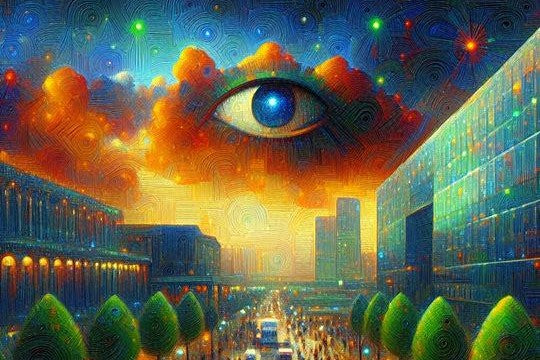
Vertu Academia: Federated Learning's Privacy-First Approach
In the age of data-driven innovation, privacy concerns have taken center stage. Enter Federated Learning, a groundbreaking paradigm in artificial intelligence that ensures collaborative model training without compromising individual privacy. This cutting-edge approach allows machines to learn from decentralized data sources without the need to share raw data, ushering in a new era of privacy-preserving AI.
Traditional AI model training often requires the centralization of vast amounts of raw data, raising legitimate concerns about privacy infringement. As the demand for intelligent systems grows, so does the need for a solution that respects user privacy while advancing the capabilities of artificial intelligence.
Federated Learning addresses the privacy challenge by enabling model training across multiple decentralized devices or servers. Instead of sending raw data to a central server, machine learning models are sent to local devices. These models learn from the data locally, and only the model updates, not the raw data, are transmitted back to the central server. This not only protects user privacy but also ensures a more inclusive and diverse dataset for training.
The key innovation lies in the decentralized nature of Federated Learning. Individual data stays on users' devices, and the collective intelligence of these devices contributes to the training of a robust and accurate model. This collaborative approach fosters a more secure and privacy-centric environment for AI development.
Advantages of Federated Learning:
-
Privacy Preservation: Users retain control over their data, and sensitive information remains on local devices. -
Inclusive Training: Federated Learning allows for diverse datasets, avoiding biases associated with centralized models. -
Efficiency: Model training occurs locally, reducing the need for large-scale data transfers and optimizing computational resources.
Challenges and Future Developments
While Federated Learning offers a promising solution to privacy concerns, challenges such as communication efficiency, model aggregation, and security must be continually addressed. Ongoing research and advancements in the field aim to make Federated Learning an even more efficient and secure approach to collaborative AI development.
No comments