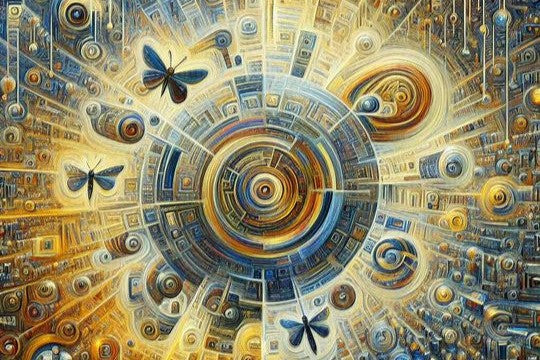
Vertu Academia: The Rise of Swarm Intelligence
Before we delve into the intricacies of Swarm Intelligence in AI, let's draw inspiration from nature. Think of a flock of birds navigating the sky or a colony of ants efficiently foraging for food. These collective behaviors are orchestrated by simple individual interactions, resulting in remarkably coordinated and intelligent group actions.
The essence of Swarm Intelligence in AI lies in replicating these collective behaviors within algorithms. Instead of relying on a single decision-maker, AI systems leverage the power of a multitude of simple entities, each following a set of rules and interacting with others. The result is a dynamic, self-organizing system capable of solving complex problems and making decisions with remarkable efficiency.
Key Principles of Swarm Intelligence in AI
Unlike traditional AI systems with a central controller, Swarm Intelligence distributes decision-making across multiple agents. Each agent, whether a virtual particle or a simulated creature, follows local rules and reacts to its environment and neighboring agents.
The true beauty of Swarm Intelligence lies in emergent intelligence—the ability of the collective to display behaviors that are more sophisticated than those of individual agents. This emergent intelligence is a result of the interactions and cooperation of the swarm's members.
AI systems employing Swarm Intelligence excel at solving complex problems that might be daunting for traditional algorithms. These swarms can tackle optimization, routing, and pattern recognition tasks with remarkable efficiency.
Applications Across Industries
The versatility of Swarm Intelligence in AI extends its applications across various industries, promising innovative solutions to longstanding challenges:
Swarm robotics, where multiple robots collaborate in a decentralized manner, is transforming automation. From warehouse logistics to search and rescue missions, swarms of robots can adapt to dynamic environments and accomplish tasks more efficiently.
Applying Swarm Intelligence to traffic management can lead to more efficient and adaptive control systems. Mimicking the flow of natural traffic, these systems can reduce congestion, enhance safety, and optimize transportation networks.
Swarm Intelligence is finding its way into financial modeling, where decentralized decision-making can be applied to predict market trends, optimize investment portfolios, and manage risk more effectively.
In healthcare, Swarm Intelligence is aiding in disease diagnosis, treatment planning, and drug discovery. AI systems, inspired by the collaborative behaviors seen in nature, are enhancing the precision and efficiency of medical decision-making.
As we conclude our exploration into the realm of Swarm Intelligence in AI, the metaphorical hive mind beckons us to appreciate the beauty of collective wisdom. From nature's intricate dance to the cutting-edge algorithms of AI, Swarm Intelligence offers a new paradigm for decision-making—one that is decentralized, adaptive, and remarkably intelligent.
No comments