Stanford Presents the Most Recent Advances in AI
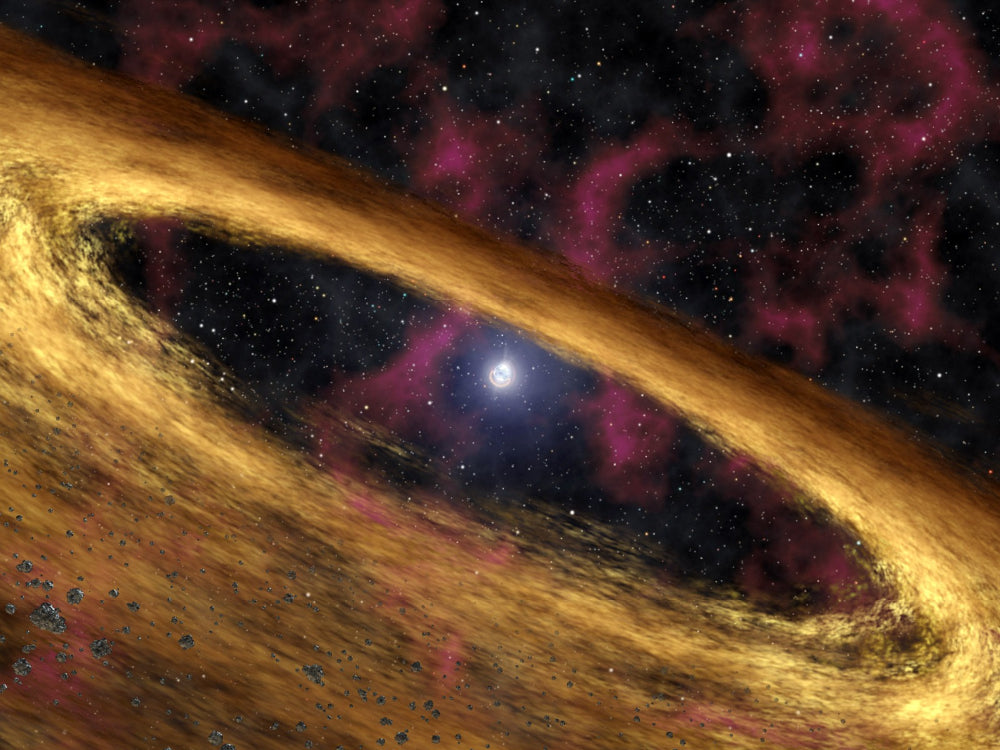
Ten years ago, the best AI systems in the world were unable to classify objects in images at a human level. AI struggled with language comprehension, much less cracking the field of mathematics. Today, AI systems are widely outperforming humans on standard benchmarks.
The Stanford HAI Institute's AI Index report is on schedule for release this year. Ray Perrault, Co-Director of the AI Index, notes that the field of AI is advancing rapidly in 2023. Tech companies are racing to build relevant products and advanced tools such as GPT-4, Gemini, and Claude 3 are delivering impressive multimodal functionality that is becoming increasingly available to the public. However, there are still significant problems with current AI technology, such as the inability to reliably process facts, perform complex reasoning, and interpret conclusions.
The 393-page AI Index 2024 report, published by the Stanford HAI Institute, provides a comprehensive overview of the latest developments in AI, including technological advances, public perceptions, and geopolitical dynamics. It also offers in-depth analysis of raw data, offering a unique insight into the current landscape.
Generative AI investment surges
While private investment in AI declined last year and overall global investment in AI fell for the second consecutive year, private investment in generative AI surged, increasing nearly eightfold from 2022 to $25.2 billion. Furthermore, the majority of private investment in generative AI is taking place in the United States.
Closed models are superior to open source models
One of the key topics in AI at the moment is the question of whether the base model should be open source or closed. Some argue that open source models are dangerous, while others say that open source models drive innovation. Rather than weighing up the arguments, the report looks at release trends and benchmark performance of each.
The number of new large-scale language models released globally in 2023 doubled from the previous year. Of the 149 base models released, 98 were open source, 23 provided partial access via APIs, and 28 were closed. While two-thirds are open source, the highest performing models come from industry participants with closed systems. Closed models outperform open source models in many common benchmarks.
Foundation models are becoming prohibitively expensive
What financial resources are required to train a large model? According to the report, the cost of AI model training has increased dramatically over time, with the cost of training advanced AI models today reaching unprecedented levels. Among them, OpenAI's GPT-4 and Google's Gemini Ultra require $78 million and $191 million in training costs, respectively.
Massive Carbon Footprint Release
It is important to consider the environmental impact of training AI models. While per-query emissions from inference may be relatively low, when models are queried thousands or even millions of times per day, the total impact can be significant.
This is because the carbon emissions data varies widely from model to model due to factors such as model size, data centre energy efficiency and carbon intensity of the energy grid. For instance, Meta's Llama 2 70B model releases approximately 291.2 tonnes of carbon, which is nearly 291 times the amount emitted by a single traveler on a round-trip flight from New York to San Francisco and 16 times the total carbon emissions of the average American in a year. Nevertheless, the Llama 2 still emits less carbon than the 502 tonnes released during OpenAI's GPT-3 training.
Google dominates the basic modelling race
In 2023, industry produced 51 notable machine learning models, while academia contributed only 15. Of these, Google released the highest number of base models. Tech companies release base models to drive advanced technology forward and to give developers a foundation on which to build products and services. Since 2019, Google has been the leader in releasing base models, with OpenAI coming in second.
